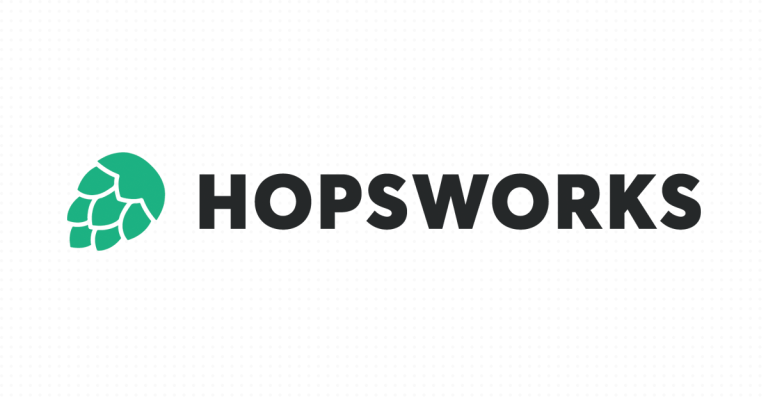
Hopsworks is a feature store and data platform designed to simplify the development and management of machine learning (ML) applications. It provides researchers and data scientists with tools to create, manage, and version features, as well as deploy models in a production environment. Hopsworks is especially well-suited for teams working on large-scale ML projects that require the integration of real-time data pipelines and feature engineering. It combines traditional data management with modern ML practices, making it a comprehensive platform for managing the entire ML lifecycle, from feature engineering to model deployment.

Modzy is an AI platform designed to make deploying, managing, and scaling machine learning models easier, with a specific focus on governance, explainability, and trust in AI systems. The platform provides tools for model deployment, monitoring, and auditing, ensuring that models can be safely and reliably used in production environments. Modzy’s emphasis on AI governance makes it particularly appealing for industries where regulations and ethical considerations are paramount, such as healthcare, finance, and government. By offering built-in tools for model explainability and bias detection, Modzy ensures that AI models are not only performant but also accountable.
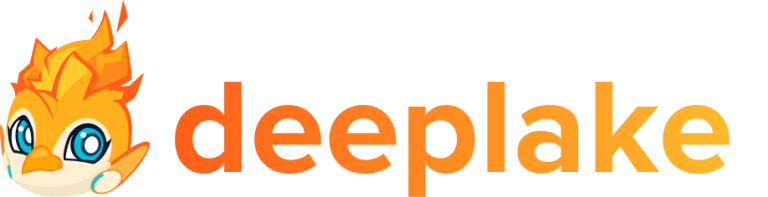
Deep Lake is a data lake specifically built for AI research and machine learning, enabling researchers to store, query, and visualize large-scale datasets efficiently. The platform focuses on optimizing dataset management for deep learning, which often involves handling massive amounts of unstructured data such as images, videos, and audio files. By providing tools to query and explore data natively and integrating seamlessly with deep learning frameworks, Deep Lake simplifies the data handling process, reducing the time spent on data wrangling and preprocessing. It’s ideal for research teams working with large datasets and in need of scalable, efficient storage and retrieval systems.
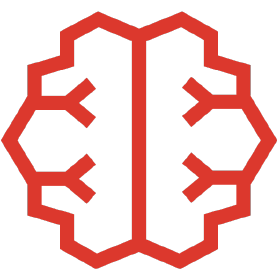
RedisAI is a platform designed for deploying and serving machine learning models at scale, using Redis as its primary database engine. It supports popular machine learning frameworks like TensorFlow, PyTorch, and ONNX, enabling researchers to deploy and serve models directly from a Redis database. RedisAI is particularly useful for real-time AI applications, where low-latency inference is critical. By integrating seamlessly with Redis, a widely used in-memory database, RedisAI ensures that models can be deployed in high-performance environments, making it ideal for research teams working on production-ready AI systems.
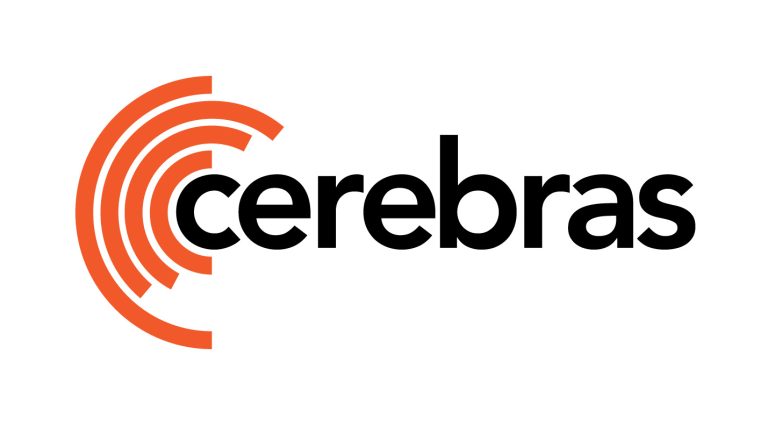
Cerebras is a high-performance AI accelerator designed to handle deep learning research at scale. At the heart of Cerebras’ technology is its Wafer-Scale Engine (WSE), the largest computer chip ever built, which is specifically optimized for AI workloads. Cerebras is designed to accelerate the training and inference of large, complex models, reducing the time and computational resources required for deep learning research. By providing cutting-edge hardware, Cerebras aims to push the boundaries of what’s possible in AI model training, making it an ideal tool for research teams working on deep neural networks and large-scale AI problems.
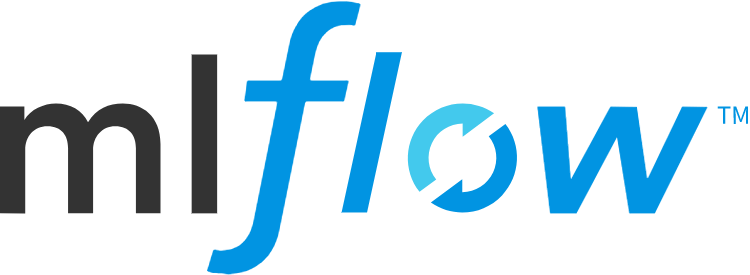
MLflow is an open-source platform designed to manage the entire machine learning lifecycle. It supports experimentation, reproducibility, and deployment of models in a streamlined and efficient manner. MLflow is a flexible and modular tool that allows users to work with any ML library, algorithm, or language. By offering tools for experiment tracking, project packaging, model management, and deployment, MLflow aims to make it easier for research teams to manage their workflows while ensuring transparency and reproducibility. It’s an excellent option for teams looking to integrate their machine learning operations seamlessly across different environments and stages of the research process.
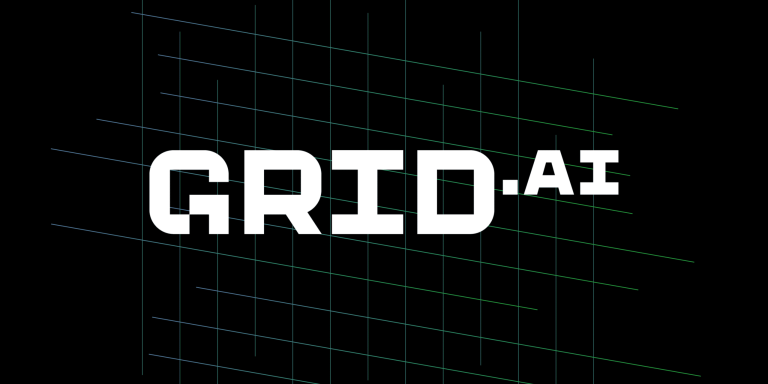
Grid.ai is a cloud platform designed to accelerate machine learning research by automating infrastructure management for training models. By using Grid.ai, researchers can easily scale their machine learning experiments across multiple GPUs or cloud instances, without having to worry about the underlying infrastructure. The platform is optimized for training deep learning models and is built on top of the PyTorch Lightning framework, making it especially valuable for research teams working with complex neural networks. Its core benefit is that it allows researchers to focus on experimentation and model development, rather than on the technical challenges of scaling and managing hardware.
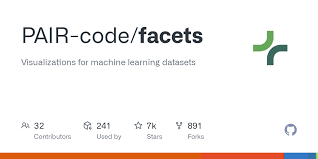
Facets is an open-source data visualization tool developed by Google, designed to help researchers and data scientists better understand the datasets they are working with for machine learning models. The tool provides rich, interactive visualizations of datasets, allowing users to explore the distribution of values, identify anomalies, and gain insights into the structure of the data. Facets offers two main components: Facets Overview, which gives a high-level summary of datasets, and Facets Dive, which allows for a more detailed, instance-level exploration of data. It is particularly useful for data preprocessing and exploratory data analysis in the early stages of machine learning research.
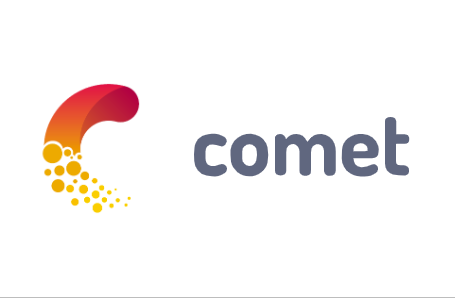
Comet.ml is a platform for tracking, managing, and optimizing machine learning experiments. It is designed to provide transparency and reproducibility across research workflows, making it easier for researchers to track the entire lifecycle of their machine learning models—from experiment setup to deployment. Comet.ml allows teams to visualize metrics, hyperparameters, datasets, and even model architectures, providing a comprehensive environment for ML experimentation. It is especially valuable for academic research environments, where reproducibility and transparency are critical, but it is also widely used in industry settings to manage complex machine learning projects.