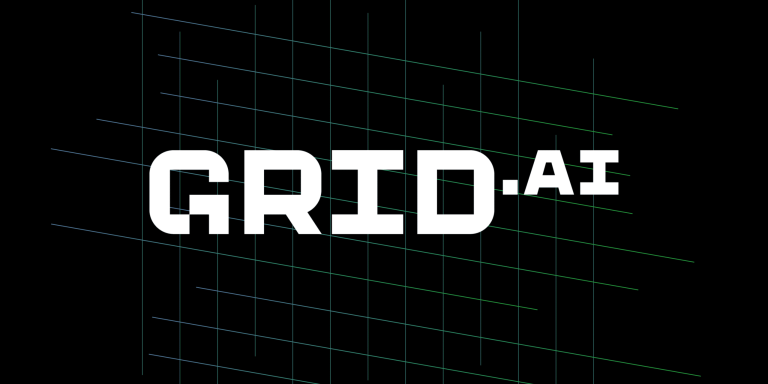
Grid.ai is a cloud platform designed to accelerate machine learning research by automating infrastructure management for training models. By using Grid.ai, researchers can easily scale their machine learning experiments across multiple GPUs or cloud instances, without having to worry about the underlying infrastructure. The platform is optimized for training deep learning models and is built on top of the PyTorch Lightning framework, making it especially valuable for research teams working with complex neural networks. Its core benefit is that it allows researchers to focus on experimentation and model development, rather than on the technical challenges of scaling and managing hardware.
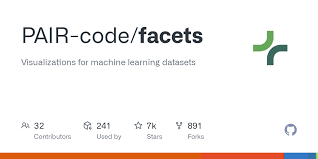
Facets is an open-source data visualization tool developed by Google, designed to help researchers and data scientists better understand the datasets they are working with for machine learning models. The tool provides rich, interactive visualizations of datasets, allowing users to explore the distribution of values, identify anomalies, and gain insights into the structure of the data. Facets offers two main components: Facets Overview, which gives a high-level summary of datasets, and Facets Dive, which allows for a more detailed, instance-level exploration of data. It is particularly useful for data preprocessing and exploratory data analysis in the early stages of machine learning research.
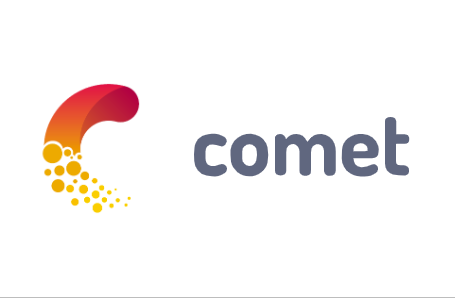
Comet.ml is a platform for tracking, managing, and optimizing machine learning experiments. It is designed to provide transparency and reproducibility across research workflows, making it easier for researchers to track the entire lifecycle of their machine learning models—from experiment setup to deployment. Comet.ml allows teams to visualize metrics, hyperparameters, datasets, and even model architectures, providing a comprehensive environment for ML experimentation. It is especially valuable for academic research environments, where reproducibility and transparency are critical, but it is also widely used in industry settings to manage complex machine learning projects.
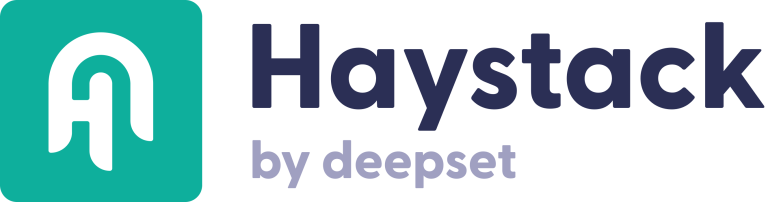
Haystack, developed by deepset AI, is an open-source natural language processing (NLP) framework that enables researchers to build search systems, question-answering applications, and document retrievers using state-of-the-art NLP models. Built on top of Hugging Face’s Transformer models, Haystack allows researchers to quickly prototype and deploy systems for neural search and question-answering. It’s especially useful for research teams working on semantic search, chatbots, and other NLP applications where fast, relevant information retrieval is necessary.
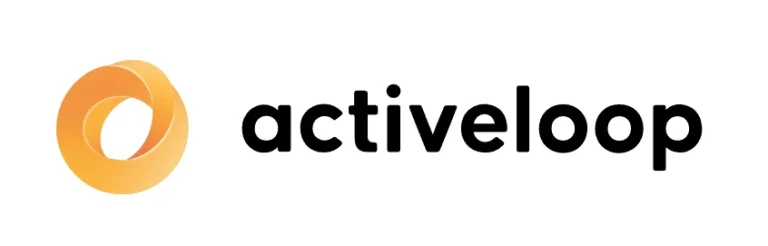
Activeloop is a platform specializing in managing and streaming large-scale datasets for machine learning, particularly in research fields like computer vision and natural language processing (NLP). One of its core features is the ability to store datasets in a format that is directly accessible for deep learning workflows, thus eliminating the need for repetitive data preprocessing tasks. Activeloop’s platform is built to handle massive datasets and stream data efficiently during training, making it highly valuable for research teams dealing with terabytes of data or those working on real-time machine learning applications.
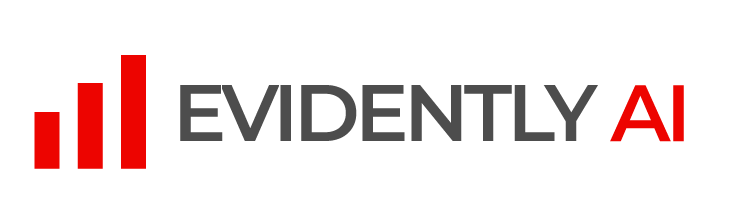
Evidently AI is a monitoring and performance tracking tool for machine learning (ML) models. It helps researchers and data scientists keep track of model performance over time, with a particular focus on identifying data drift, performance degradation, and bias issues. Designed to enhance transparency and trust in machine learning systems, Evidently AI enables researchers to generate in-depth reports on their models and understand when models might need retraining or adjustment. This tool is especially valuable for those who want to ensure their models perform well in real-world settings where the data distribution may change over time.
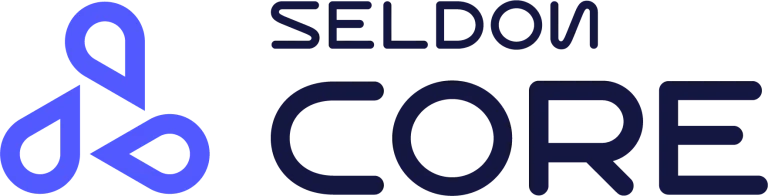
Seldon is a machine learning deployment and monitoring platform designed to focus on explainability, fairness, and real-time inference. It provides the infrastructure to deploy machine learning models in production environments with ease, while offering insights into how these models perform, especially with regard to explainability and fairness. For researchers working on applied machine learning projects or teams deploying models in sensitive fields, such as healthcare or finance, Seldon ensures transparency and accountability in machine learning workflows.
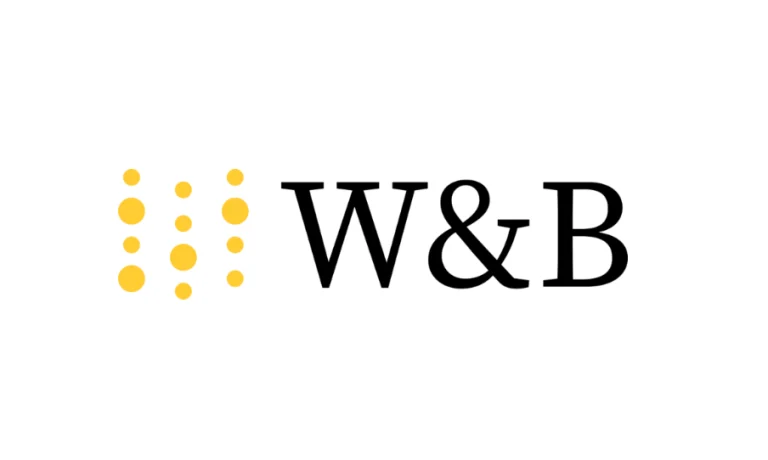
Weights & Biases (W&B) is a platform designed to help machine learning teams track experiments, version datasets, monitor models in real-time, and optimize hyperparameters. It provides tools for visualizing machine learning workflows, which is essential for keeping research organized and ensuring the reproducibility of experiments. With its focus on experiment tracking, W&B allows researchers to compare models, visualize results, and streamline collaborative work across teams. Its compatibility with a wide range of machine learning frameworks makes it versatile for researchers using different approaches.

Gradio is a Python library designed to create easy-to-use graphical interfaces for machine learning models, making it possible for researchers and developers to quickly prototype and test models with minimal effort. Whether you're looking to create an interactive web application or share your machine learning model for public testing, Gradio simplifies the process of building customizable UIs without extensive web development knowledge. It’s especially useful for testing, sharing, and gathering feedback on models, offering researchers a way to quickly deploy and demonstrate their AI work.